注意
转到末尾下载完整示例代码。或通过 Binder 在浏览器中运行此示例
为灰度图像着色#
用某种颜色人为地为图像着色可能很有用,无论是突出图像的特定区域,还是仅仅是活跃灰度图像。此示例演示了通过缩放 RGB 值以及在 HSV 色彩空间中调整颜色来为图像着色。
在 2D 中,彩色图像通常以 RGB 表示 - 2D 数组的 3 层,其中 3 层表示图像的(R)红色、(G)绿色和(B)蓝色通道。获得着色图像的最简单方法是将每个 RGB 通道设置为灰度图像,该灰度图像乘以每个通道的不同乘数。例如,将绿色和蓝色通道乘以 0 只留下红色通道,并产生明亮的红色图像。同样,将蓝色通道归零只留下红色和绿色通道,它们组合起来形成黄色。
import matplotlib.pyplot as plt
from skimage import data
from skimage import color
from skimage import img_as_float, img_as_ubyte
grayscale_image = img_as_float(data.camera()[::2, ::2])
image = color.gray2rgb(grayscale_image)
red_multiplier = [1, 0, 0]
yellow_multiplier = [1, 1, 0]
fig, (ax1, ax2) = plt.subplots(ncols=2, figsize=(8, 4), sharex=True, sharey=True)
ax1.imshow(red_multiplier * image)
ax2.imshow(yellow_multiplier * image)
plt.show()
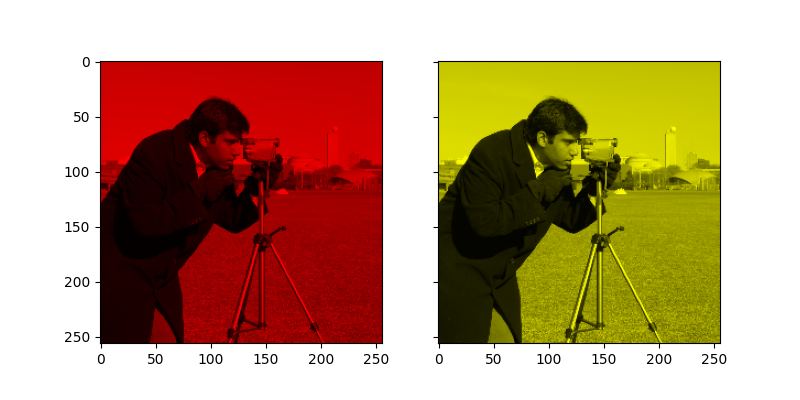
在许多情况下,处理 RGB 值可能不是理想的。因此,有许多其他的 色彩空间 可以用来表示彩色图像。一种流行的色彩空间称为 HSV,它表示色调(〜颜色)、饱和度(〜色彩度)和值(〜亮度)。例如,一种颜色(色调)可能是绿色,但其饱和度是该绿色的强度 - 其中橄榄色在低端,霓虹色在高端。
在某些实现中,HSV 中的色调从 0 到 360,因为色调在一个圆圈中环绕。然而,在 scikit-image 中,色调是 0 到 1 的浮点值,因此色调、饱和度和值都共享相同的比例。
下面,我们绘制色调中的线性渐变,饱和度和值都调到最大
import numpy as np
hue_gradient = np.linspace(0, 1)
hsv = np.ones(shape=(1, len(hue_gradient), 3), dtype=float)
hsv[:, :, 0] = hue_gradient
all_hues = color.hsv2rgb(hsv)
fig, ax = plt.subplots(figsize=(5, 2))
# Set image extent so hues go from 0 to 1 and the image is a nice aspect ratio.
ax.imshow(
all_hues, extent=(0 - 0.5 / len(hue_gradient), 1 + 0.5 / len(hue_gradient), 0, 0.2)
)
ax.set_axis_off()
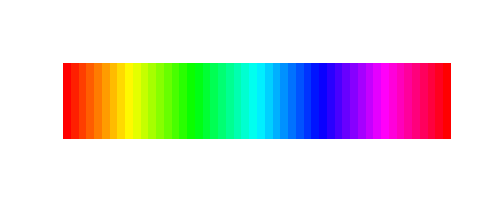
请注意,最左侧和最右侧的颜色是相同的。这反映了色调像色轮一样环绕的事实(有关更多信息,请参阅 HSV)。
现在,让我们创建一个小实用函数来获取 RGB 图像,然后
1. 将 RGB 图像转换为 HSV 2. 设置色调和饱和度 3. 将 HSV 图像转换回 RGB
def colorize(image, hue, saturation=1):
"""Add color of the given hue to an RGB image.
By default, set the saturation to 1 so that the colors pop!
"""
hsv = color.rgb2hsv(image)
hsv[:, :, 1] = saturation
hsv[:, :, 0] = hue
return color.hsv2rgb(hsv)
请注意,我们需要提高饱和度;饱和度为零的图像是灰度图像,因此我们需要一个非零值才能实际看到我们设置的颜色。
使用上面的函数,我们绘制六个具有色调线性渐变和非零饱和度的图像
hue_rotations = np.linspace(0, 1, 6)
fig, axes = plt.subplots(nrows=2, ncols=3, sharex=True, sharey=True)
for ax, hue in zip(axes.flat, hue_rotations):
# Turn down the saturation to give it that vintage look.
tinted_image = colorize(image, hue, saturation=0.3)
ax.imshow(tinted_image, vmin=0, vmax=1)
ax.set_axis_off()
fig.tight_layout()
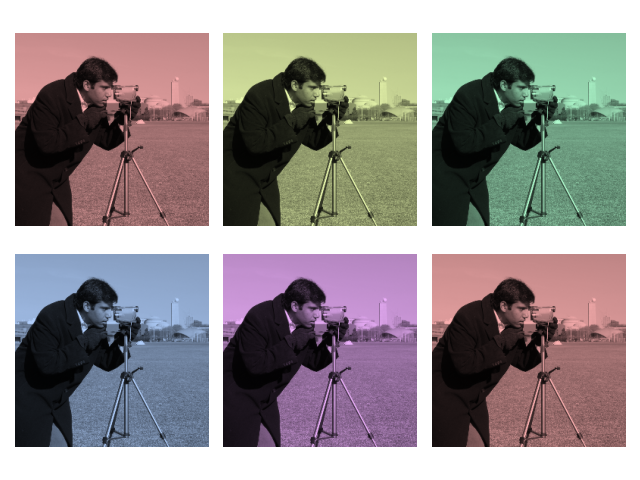
您可以将这种着色效果与 numpy 切片和花式索引结合使用,以选择性地为图像着色。在下面的示例中,我们使用切片设置某些矩形的色调,并缩放通过阈值处理找到的一些像素的 RGB 值。在实践中,您可能希望基于分割结果或斑点检测方法来定义着色的区域。
from skimage.filters import rank
# Square regions defined as slices over the first two dimensions.
top_left = (slice(25),) * 2
bottom_right = (slice(-25, None),) * 2
sliced_image = image.copy()
sliced_image[top_left] = colorize(image[top_left], 0.82, saturation=0.5)
sliced_image[bottom_right] = colorize(image[bottom_right], 0.5, saturation=0.5)
# Create a mask selecting regions with interesting texture.
noisy = rank.entropy(img_as_ubyte(grayscale_image), np.ones((9, 9)))
textured_regions = noisy > 4.25
# Note that using `colorize` here is a bit more difficult, since `rgb2hsv`
# expects an RGB image (height x width x channel), but fancy-indexing returns
# a set of RGB pixels (# pixels x channel).
masked_image = image.copy()
masked_image[textured_regions, :] *= red_multiplier
fig, (ax1, ax2) = plt.subplots(
ncols=2, nrows=1, figsize=(8, 4), sharex=True, sharey=True
)
ax1.imshow(sliced_image)
ax2.imshow(masked_image)
plt.show()
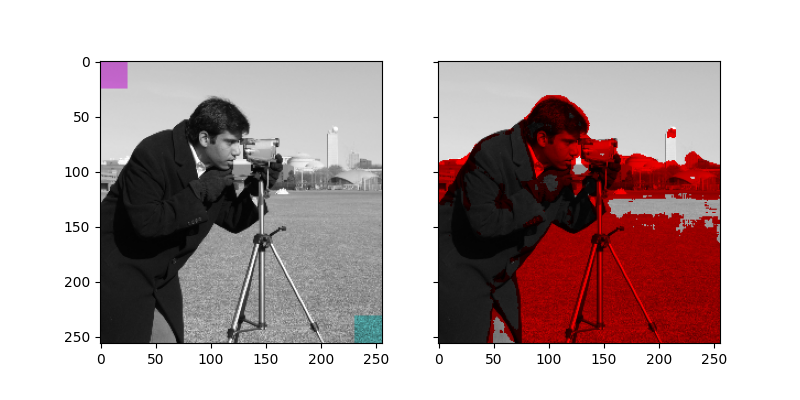
对于为多个区域着色,您可能还对 skimage.color.label2rgb 感兴趣。
脚本的总运行时间:(0 分钟 2.700 秒)