注意
跳转到末尾以下载完整的示例代码。或通过 Binder 在浏览器中运行此示例
Niblack 和 Sauvola 阈值化#
Niblack 和 Sauvola 阈值是局部阈值技术,对于背景不均匀的图像非常有用,尤其是对于文本识别 [1], [2]。不是为整个图像计算单个全局阈值,而是通过使用特定的公式,考虑局部邻域(由围绕像素的窗口定义)的平均值和标准偏差,为每个像素计算多个阈值。
在此,我们使用这些算法对图像进行二值化,并将其与常见的全局阈值技术进行比较。参数 window_size
决定了包含周围像素的窗口的大小。
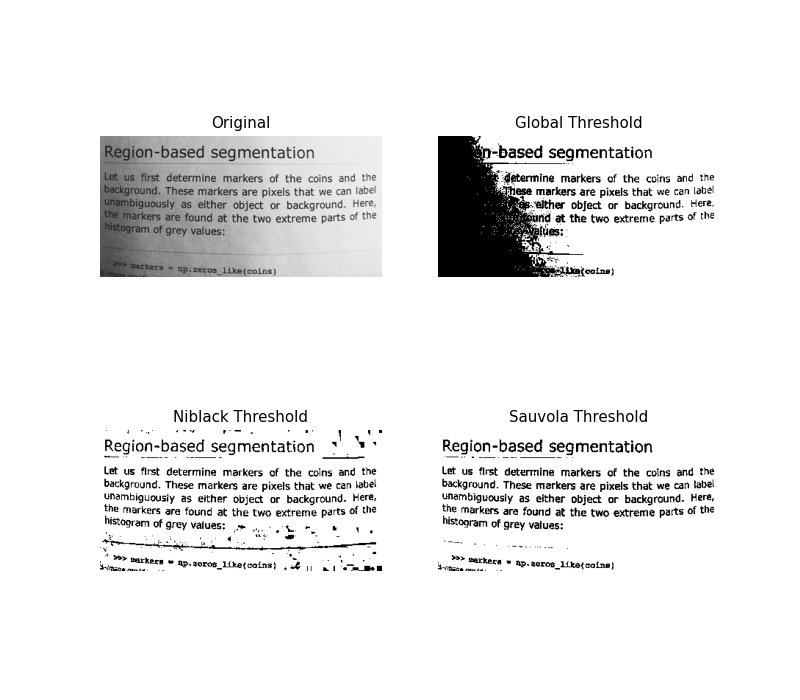
import matplotlib
import matplotlib.pyplot as plt
from skimage.data import page
from skimage.filters import threshold_otsu, threshold_niblack, threshold_sauvola
matplotlib.rcParams['font.size'] = 9
image = page()
binary_global = image > threshold_otsu(image)
window_size = 25
thresh_niblack = threshold_niblack(image, window_size=window_size, k=0.8)
thresh_sauvola = threshold_sauvola(image, window_size=window_size)
binary_niblack = image > thresh_niblack
binary_sauvola = image > thresh_sauvola
plt.figure(figsize=(8, 7))
plt.subplot(2, 2, 1)
plt.imshow(image, cmap=plt.cm.gray)
plt.title('Original')
plt.axis('off')
plt.subplot(2, 2, 2)
plt.title('Global Threshold')
plt.imshow(binary_global, cmap=plt.cm.gray)
plt.axis('off')
plt.subplot(2, 2, 3)
plt.imshow(binary_niblack, cmap=plt.cm.gray)
plt.title('Niblack Threshold')
plt.axis('off')
plt.subplot(2, 2, 4)
plt.imshow(binary_sauvola, cmap=plt.cm.gray)
plt.title('Sauvola Threshold')
plt.axis('off')
plt.show()
脚本的总运行时间:(0 分钟 0.347 秒)