注意
转到末尾以下载完整的示例代码。 或者通过 Binder 在浏览器中运行此示例
测量核膜处的荧光强度#
此示例重现了生物图像数据分析中用于测量定位于核膜的荧光强度的成熟工作流程,在细胞图像的时间序列(每个图像具有两个通道和两个空间维度)中,该序列显示了蛋白质从细胞质区域重新定位到核膜的过程。此生物学应用最初由 Andrea Boni 和合作者在 [1] 中提出;它被 Kota Miura 在教科书中 [2] 以及其他作品 ([3], [4]) 中使用。换句话说,我们将此工作流程从 ImageJ Macro 移植到使用 scikit-image 的 Python。
import matplotlib.pyplot as plt
import numpy as np
import plotly.io
import plotly.express as px
from scipy import ndimage as ndi
from skimage import filters, measure, morphology, segmentation
from skimage.data import protein_transport
我们从单个细胞/细胞核开始构建工作流程。
image_sequence = protein_transport()
print(f'shape: {image_sequence.shape}')
shape: (15, 2, 180, 183)
该数据集是一个 2D 图像堆栈,包含 15 帧(时间点)和 2 个通道。
vmin, vmax = 0, image_sequence.max()
fig = px.imshow(
image_sequence,
facet_col=1,
animation_frame=0,
zmin=vmin,
zmax=vmax,
binary_string=True,
labels={'animation_frame': 'time point', 'facet_col': 'channel'},
)
plotly.io.show(fig)
首先,让我们考虑第一个图像的第一个通道(下图中的步骤 a)
)。
image_t_0_channel_0 = image_sequence[0, 0, :, :]
分割细胞核边缘#
让我们将高斯低通滤波器应用于此图像以使其平滑(步骤 b)
)。接下来,我们分割细胞核,使用 Otsu 方法找到背景和前景之间的阈值:我们得到一个二值图像(步骤 c)
)。然后,我们填充对象中的孔洞(步骤 c-1)
)。
smooth = filters.gaussian(image_t_0_channel_0, sigma=1.5)
thresh_value = filters.threshold_otsu(smooth)
thresh = smooth > thresh_value
fill = ndi.binary_fill_holes(thresh)
按照原始工作流程,让我们移除接触图像边界的对象(步骤 c-2)
)。在这里,我们可以看到另一个细胞核的一部分接触了右下角。
dtype('bool')
我们计算此二值图像的形态学膨胀(步骤 d)
)及其形态学腐蚀(步骤 e)
)。
最后,我们从膨胀中减去腐蚀以获得细胞核边缘(步骤 f)
)。这等效于选择 dilate
中但不在 erode
中的像素
mask = np.logical_and(dilate, ~erode)
让我们在子图序列中可视化这些处理步骤。
fig, ax = plt.subplots(2, 4, figsize=(12, 6), sharey=True)
ax[0, 0].imshow(image_t_0_channel_0, cmap=plt.cm.gray)
ax[0, 0].set_title('a) Raw')
ax[0, 1].imshow(smooth, cmap=plt.cm.gray)
ax[0, 1].set_title('b) Blur')
ax[0, 2].imshow(thresh, cmap=plt.cm.gray)
ax[0, 2].set_title('c) Threshold')
ax[0, 3].imshow(fill, cmap=plt.cm.gray)
ax[0, 3].set_title('c-1) Fill in')
ax[1, 0].imshow(clear, cmap=plt.cm.gray)
ax[1, 0].set_title('c-2) Keep one nucleus')
ax[1, 1].imshow(dilate, cmap=plt.cm.gray)
ax[1, 1].set_title('d) Dilate')
ax[1, 2].imshow(erode, cmap=plt.cm.gray)
ax[1, 2].set_title('e) Erode')
ax[1, 3].imshow(mask, cmap=plt.cm.gray)
ax[1, 3].set_title('f) Nucleus Rim')
for a in ax.ravel():
a.set_axis_off()
fig.tight_layout()
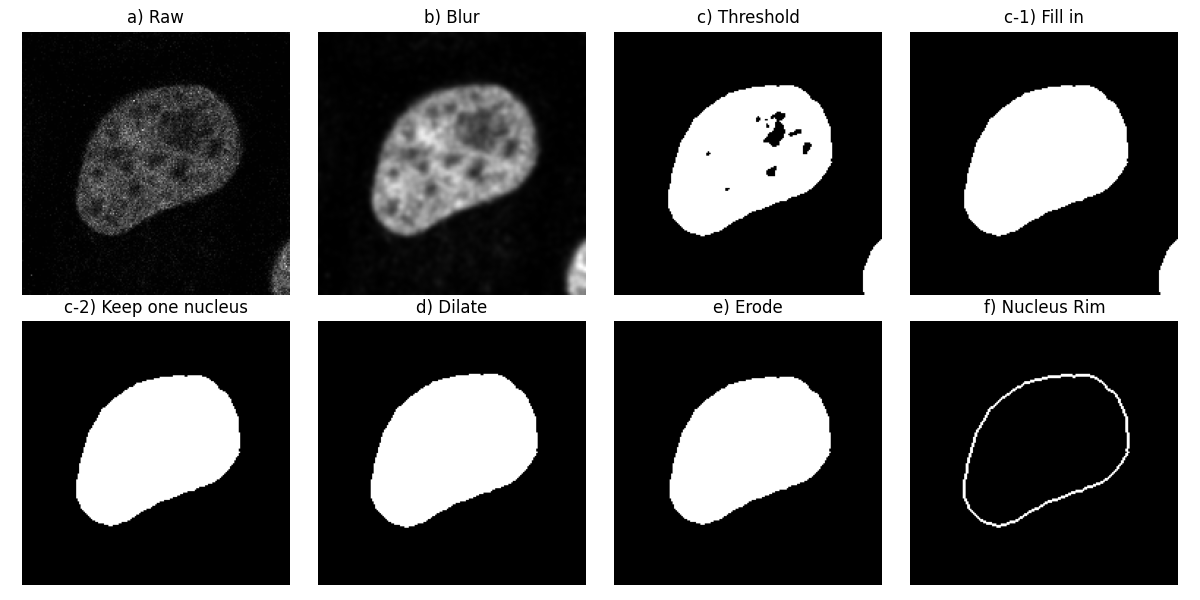
将分割的边缘应用为掩码#
现在我们已经分割了第一个通道中的核膜,我们将其用作掩码来测量第二个通道中的强度。
image_t_0_channel_1 = image_sequence[0, 1, :, :]
selection = np.where(mask, image_t_0_channel_1, 0)
fig, (ax0, ax1) = plt.subplots(1, 2, figsize=(12, 6), sharey=True)
ax0.imshow(image_t_0_channel_1)
ax0.set_title('Second channel (raw)')
ax0.set_axis_off()
ax1.imshow(selection)
ax1.set_title('Selection')
ax1.set_axis_off()
fig.tight_layout()
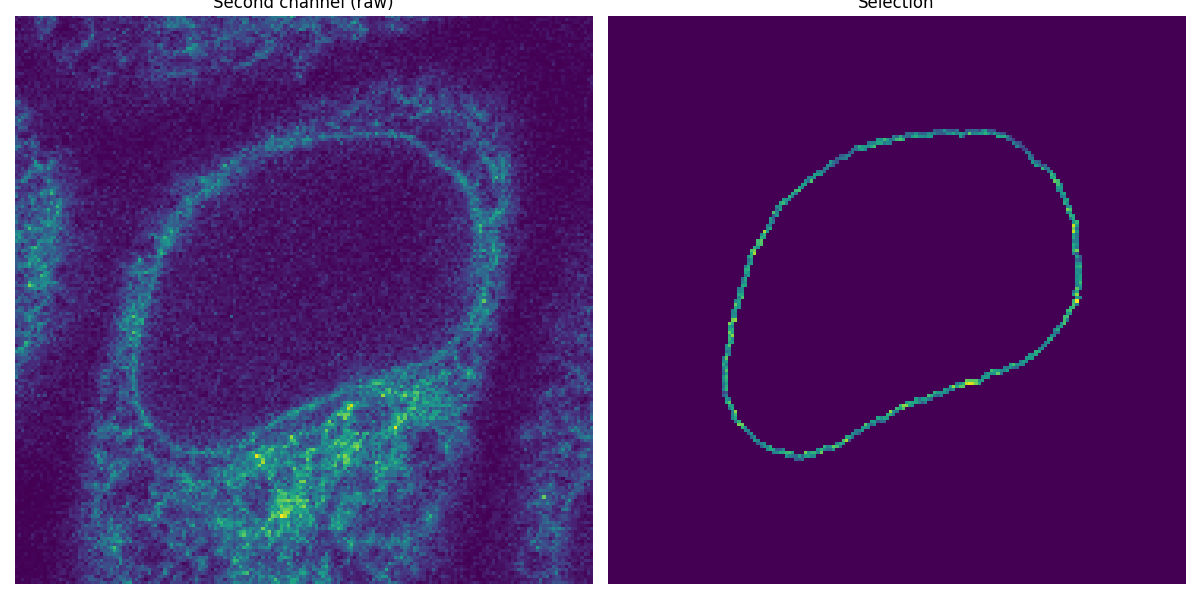